When cleansing B2B data the risks of concentrating on the wrong things are real.
Many data cleansing vendors are setup primarily for B2C.
These vendors will concentrate on the large numbers of contacts and potentially inaccurate phone numbers, emails etc. The commercial attractions of charging per contact for this kind of cleansing are obvious.
But whilst contacts probably are the centre of the B2C universe, for B2B they aren’t. The company identity is even more important. Getting contact names right is pointless if you shouldn’t be pursuing that company as a prospect.
Standard Automated Data retrieval from Companies House
This appears attractive. A standard service “just” does it.
But a standard service or API that “just does” is likely to assume you have accurate data. So the company name entry will match exactly etc. etc.
But hang on. Your CRM data is imperfect. This what we’re trying to fix! There will be gaps, typos etc. So one can wind up people spending time cleansing B2B data either before or after a standardised retrieval.
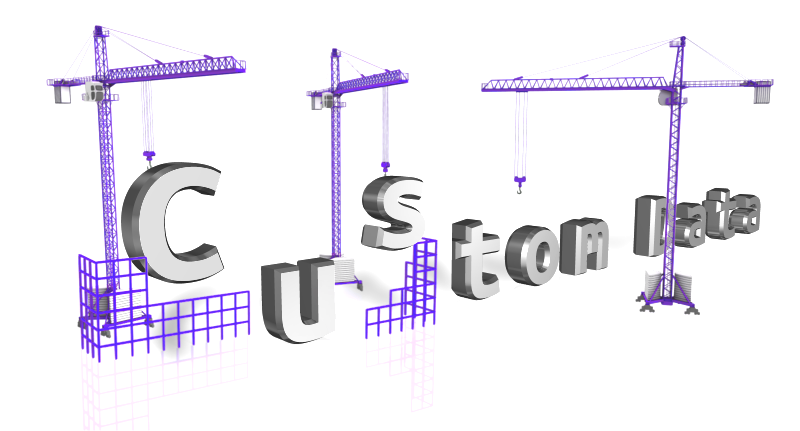
“Specific Features” – added for good reason
But as well imperfect your CRM data may have specific features. People may have applied particular tactics to workaround what they saw as problems. For instance people may have applied prefixes or suffixes to help with deduplication. So any “generic” solution could struggle to handle this data.
In other cases people may just have “particular ways” or encountered peculiar nasties that have affected the data. So any real solution must cope with these.
The peregrine approach is different
We assume your input CRM data may be far from perfect. So we’ve designed our system to accept poor data, whether through APIs or manual entry.
We assume your customer data can have “specific features”. So our system can apply “transforms” built for just your situation. So the system is built ground up to allow per client customisation.
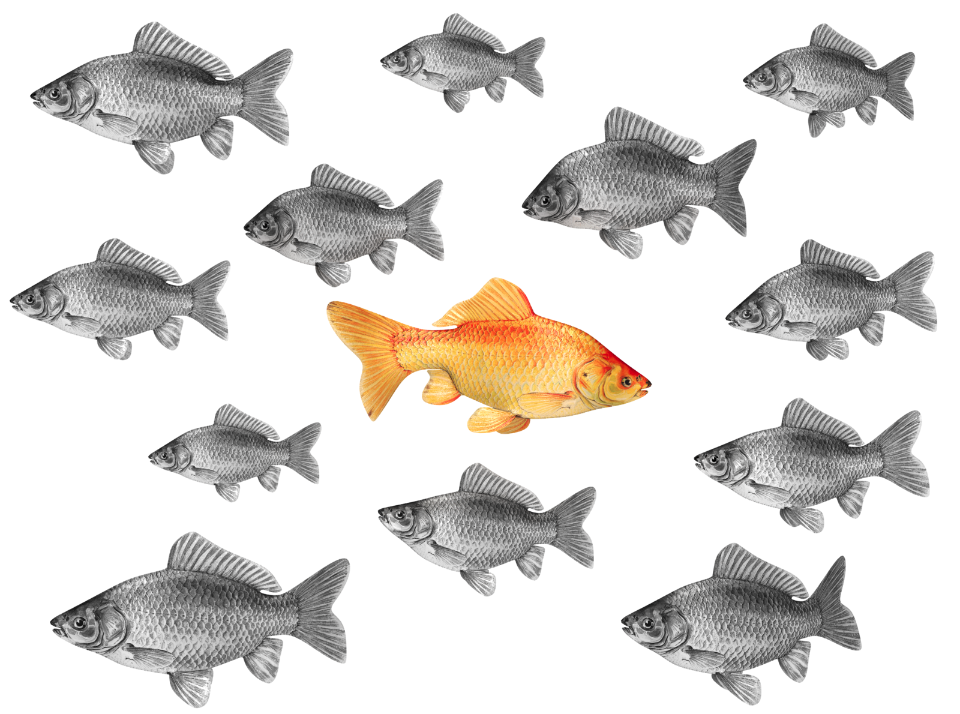
We assume that given “fuzzy data input” we should generate a list of candidate results. And provide supporting information that makes it possible automatically or otherwise to pick the best candidate.
We assume that historic data will need to be cleaned up. This may be huge in scope – and that developing our process(es) to help constrain the supporting staffing costs in your organisation is inherently sensible.
We assume that numeric data and facts should be pushed into your CRM database – facilitating automated downstream reporting and analysis.